- Computing power annecdotally doubles every two years.
- Machine learning and artificial intelligence require much more power.
- Nvidia has developed powerful graphic processing units that execute tasks in parallel.
- They have a first mover advantage in machine learning and Artificial Intelligence
Moore's law refers to an observation made by Intel co-founder Gordon Moore.
In 1965, Moore saw that transistors were shrinking so fast that every year twice as many could fit onto a chip. Ten years later, he adjusted the pace of his theory to a doubling every two years.
Essentially, Moore’s law indicated that the power of computing would double roughly every two years.
Most PCs or servers contain a microprocessor. These are known as a “central processing unit” (CPU) which can deal with most workloads (the amount of processing the computer has been given to do at a given time). It just so happens, the company Moore founded made the most powerful CPUs and have ruled the market for PC processors ever since. The Nasdaq-listed Nvidia currently controls around 80% market share.
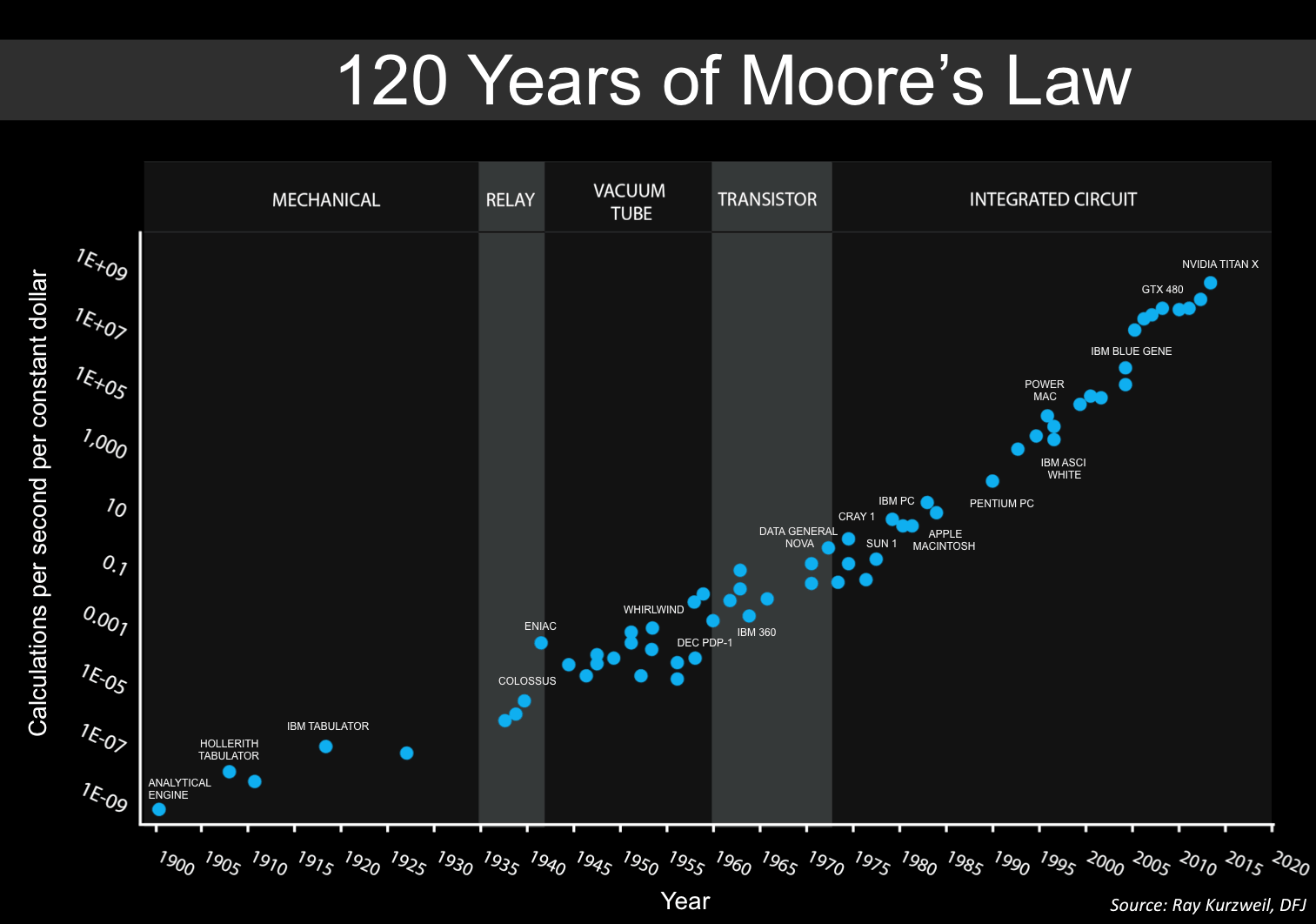
That said, despite its official-sounding name, Moore’s Law is not a scientific rule of law like Newton’s laws of motion.
It only really describes the rapid pace at which a change in the manufacturing process has made computers exponentially more affordable and available to the average consumer.
However, Moore’s law is reaching a limit as processors are struggling to handle some of the more complex tasks being asked of them. Things like machine learning and other Artificial Intelligence applications require vast amounts of data and consume more power than entire data centres did only a few years ago. Chips weren't evolving fast enough.
This has opened the door for more specialised chips that would work alongside Intel processes and squeeze more benefits from the application of Moore’s law. Big data centres are opting for more and more specialised processors from other companies such as Nvidia's graphics processing units (GPU’s). Initially the calling card of serious gamers everywhere, these chips were created to carry out the massive and complex computation that is required by interactive video games.
Nvidia’s GPU’s have hundreds of specialised cores or brains that generate triangles to form frame-like structures, simulating objects and applying colours to the pixel on a display screen. To do that, many simple instructions must be executed in parallel. These brains in the GPU allowing it to handle complex artificial intelligence tasks like image, facial and speech recognition. All of a sudden, every online giant is using Nvidia GPUs to give their AI services the capability to ingest reams of data.
So, CPU is kind of like a swiss army knife. It’s able to handle lots of random and diverse instructions efficiently. This allows you to multitask on your computer efficiently, and means your computer is very good at doing lots of things very quickly. Whereas, GPUs were traditionally there for one purpose to render graphics. This unity of purpose involved doing millions of very similar calculations at the same time in parallel. Because of this, they have been focused more on raw throughput. The GPU is more like a scalpel.
Excitement about AI applications has rapidly changed the fortunes of Nvidia. Its stock-market value has ballooned more than sevenfold in the past two years, topping $US100 billion, and its revenue jumped 56% in the most recent quarter. Nvidia shares have rallied nearly 190% during the previous 12 months.
We’re only just beginning to scratch the surface of Artificial Intelligence and machine learning. There’s going to be more and more data and applications that we hadn’t even thought of. Nvidia has put itself in the box seat with the AI revolution. It has the first mover advantage and the ability to spend more than its rivals on AI chips.
At Spaceship, we’re excited about the future of AI and think that’s where the world is going. That’s why we have exposure to Nvidia in our superannuation portfolio.